Predictive Analytics and Decision-Making: Transforming Business Through Data-Driven Insights.
- kapilramjattan
- Mar 24
- 8 min read
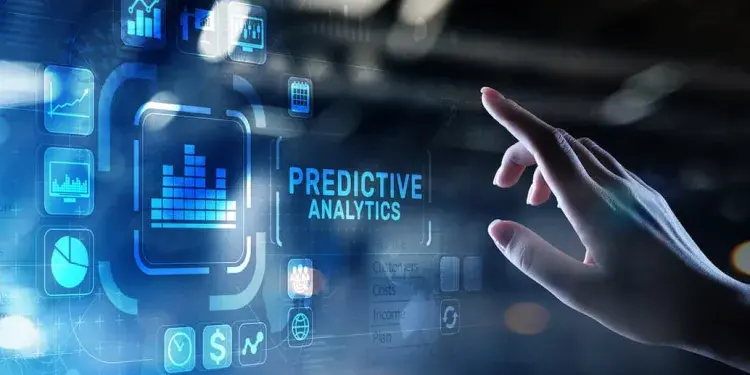
In today's data-rich business environment, the ability to anticipate future outcomes has become a critical competitive advantage. Predictive analytics stands at the forefront of this revolution, empowering organizations to move beyond reactive decision-making to a more proactive approach that anticipates challenges and opportunities before they arise. This article explores the fundamentals of predictive analytics, its techniques, applications across industries, benefits, challenges, and future trends.
Understanding Predictive Analytics
Predictive analytics is an advanced form of data analytics that attempts to answer the question, "What might happen next?" Unlike descriptive analytics that explains what has happened or diagnostic analytics that explores why something happened, predictive analytics looks forward, using historical data, statistical algorithms, and machine learning techniques to identify the likelihood of future outcomes.
As a branch of data science for business, predictive analytics has grown alongside big data systems. Larger, broader pools of data enable increased data mining activities to provide predictive insights, while advancements in big data machine learning have expanded predictive analytics capabilities.
Other terms often used interchangeably with predictive analytics include forecasting analytics, predictive modeling, data forecasting, and predictive insights. While these terms share similarities, predictive analytics encompasses the broader practice of using data to make predictions about future events or behaviors.
How Predictive Analytics Works
The predictive analytics process typically follows these key steps:
Data collection: Gathering relevant historical data from various sources, including internal databases, external datasets, and real-time data streams.
Data cleaning: Removing inconsistencies, handling missing values, and preparing the data for analysis. This critical step ensures the quality of predictions.
Analysis: Applying statistical techniques and algorithms to identify patterns, correlations, and trends within the data.
Modeling: Creating predictive models based on the patterns identified. These models establish relationships between variables to predict future outcomes.
Validation: Testing the models against known outcomes to ensure accuracy and reliability.
Deployment: Implementing the models to make predictions in real-world scenarios.
Monitoring: Continuously evaluating and refining the models to maintain or improve their predictive power as new data becomes available.
Types of Predictive Analytics Models
There are two general types of predictive analytics models:
Classification models attempt to categorize data objects (such as customers or potential outcomes) into distinct groups. For instance, a retailer might use classification models to predict which types of customers will be receptive to marketing emails or which transactions might be fraudulent.
Regression models predict continuous data, such as how much revenue a customer will generate during their relationship with the company, how many units of a product will sell next quarter, or how prices might change in response to market conditions.
Key Predictive Analytics Techniques
Regression Analysis
Regression analysis is a statistical technique that estimates relationships between variables. It is particularly useful for determining patterns in large datasets and understanding the correlation between inputs. Regression works best with continuous data that follows a known distribution.
Types of regression include:
Linear regression: Models the relationship between a dependent variable and one or more independent variables using a linear equation
Logistic regression: Used for binary classification problems
Multiple regression: Analyzes the relationship between multiple independent variables and one dependent variable
A common application of regression analysis is determining how one or more independent variables affect another, such as how a price increase will affect the sale of a product.
Decision Trees
Decision trees are classification models that place data into different categories based on distinct variables. The model resembles a tree, with each branch representing a potential choice and each leaf representing the result of that decision.
Decision trees excel at helping understand individual decisions and are typically easy to interpret, making them valuable when you need to make decisions quickly. They also work well when a dataset has several missing variables, as they can accommodate incomplete information better than some other techniques.
Neural Networks
Neural networks are machine learning methods that excel at modeling very complex relationships. These powerful pattern recognition engines can identify nonlinear relationships in datasets, especially when no known mathematical formula exists to analyze the data.
Part of the emerging field of deep learning, neural networks can validate the results of decision trees and regression models. They're particularly valuable for complex tasks like image recognition, natural language processing, and other applications where traditional statistical methods might fall short.
Applications Across Industries
Predictive analytics is transforming decision-making across various sectors:
Finance and Banking
Financial institutions leverage predictive analytics for:
Credit scoring: Assessing the risk that a borrower might fail to repay a loan based on credit history and similar borrowers' characteristics
Fraud detection: Examining transactions for irregular patterns that might indicate fraudulent activity
Market trading: Guiding investment decisions and portfolio management based on historical market data and trends
Risk assessment: Predicting extreme events to mitigate insurance claims and manage financial risk
Retail and E-Commerce
Retailers use predictive analytics to:
Demand forecasting: Predict which products will be in high demand during certain seasons
Inventory management: Pre-deliver stock to regional and local distribution stations to reduce delivery times
Personalized recommendations: Increase up- and cross-selling opportunities based on customer profiles
Lead scoring: Improve conversion rates by identifying the most promising prospects
Manufacturing
Manufacturers apply predictive analytics for:
Predictive maintenance: Predict when equipment will need maintenance to prevent breakdowns
Quality control: Detect irregularities and highlight equipment defects before they cause major issues
Supply chain optimization: Forecast disruptions and adjust shipping and inventory plans
Production optimization: Monitor production line equipment to maximize throughput and efficiency
Healthcare
Healthcare providers utilize predictive analytics for:
Patient monitoring: Detect real-time changes in patients' conditions while eliminating spurious alarms
Disease prediction: Forecast disease progression based on patient data and medical history
Resource allocation: Optimize hospital resources and staff scheduling
Treatment planning: Predict the effectiveness of different treatment options for individual patients
Benefits of Predictive Analytics
Organizations implementing predictive analytics can realize numerous advantages:
Improved decision-making: Data-driven insights guide strategic decisions and reduce reliance on intuition or guesswork. This leads to more consistent and objective decision-making across the organization.
Increased accuracy: Predictions based on data are typically more reliable than traditional guesswork or subjective assessments, especially when dealing with complex systems or large amounts of information.
Cost savings: By optimizing processes and preventing issues before they occur, organizations can significantly reduce operational costs. Predictive maintenance alone can save manufacturing companies millions by preventing unexpected downtime.
Better risk management: Early warning signs allow businesses to take action before issues become major problems. This proactive approach to risk can prevent financial losses and reputation damage.
Enhanced customer satisfaction: Through personalized services and improved experiences based on customer preferences and behavior patterns, businesses can increase loyalty and retention.
Competitive advantage: Companies using predictive analytics can stay ahead of competitors by anticipating market trends and customer needs before they become obvious to everyone in the industry.
Operational efficiency: Streamlining operations and resource allocation based on predicted needs and demands leads to better utilization of assets and personnel.
Proactive problem-solving: Addressing issues before they impact the business, rather than reacting after problems occur, minimizes disruption and maintains productivity.
Challenges in Implementing Predictive Analytics
Despite its potential, organizations face several challenges when implementing predictive analytics:
Data quality: Accurate predictions depend on good-quality data. Incomplete, inaccurate, or biased data can lead to poor results and misleading predictions that may cause more harm than good.
Model complexity: Building and maintaining predictive models requires specialized expertise. The more complex the model, the more difficult it can be to develop, understand, and maintain.
Privacy concerns: Using personal data raises privacy issues, especially in sectors like healthcare and finance. Organizations must navigate data protection laws and ethical considerations when collecting and analyzing data.
Implementation costs: Deploying predictive analytics systems can be expensive, particularly for smaller businesses with limited resources. The investment includes not just technology but also skilled personnel and organizational change management.
Interpretation challenges: Understanding and explaining complex models can be difficult, especially when communicating results to non-technical stakeholders who need to make decisions based on the predictions.
Changing patterns: Historical patterns may not always predict future events, especially during unusual circumstances or rapid market changes. Models need regular updating to remain relevant.
Predictive Analytics vs. Machine Learning
A common misconception is that predictive analytics and machine learning are synonymous. While they're related, they represent different concepts:
Predictive analytics is the broader practice of using data to make predictions about future events or behaviors. It encompasses various techniques, including statistical methods, data mining, and machine learning.
Machine learning is a specific approach within artificial intelligence that focuses on developing algorithms that can learn from and make predictions based on data. It's one of the tools used in predictive analytics, but not the only one.
In essence, machine learning is a means to achieve predictive analytics, while predictive analytics is the overall goal of forecasting future outcomes based on historical data.
The Future of Predictive Analytics
The future of predictive analytics looks promising with several trends emerging:
Integration with AI: Deeper integration with artificial intelligence will create more sophisticated predictive models that can handle increasingly complex data and scenarios, leading to more accurate and nuanced predictions.
Democratization: Predictive analytics tools are becoming more accessible to non-technical users through user-friendly interfaces and automated model building. This trend will continue, allowing more organizations to benefit from predictive capabilities.
Real-time analytics: Moving from batch processing to real-time predictive insights will enable immediate decision-making based on current data, critical for time-sensitive applications like fraud detection or dynamic pricing.
Edge computing: Processing data closer to where it's generated will enable faster predictions, reducing latency and enabling more responsive systems, particularly important for Internet of Things (IoT) applications.
Explainable AI: As predictive models become more complex, the development of techniques that can explain their predictions in human-understandable terms will increase trust and adoption, especially in regulated industries.
Automated machine learning: Automating the process of building and deploying predictive models will make it faster and more accessible, reducing the need for specialized data science expertise for basic predictive tasks.
Emerging Applications
As predictive analytics technology advances, new applications are emerging:
Personalized medicine: Tailoring medical treatments to individual characteristics of each patient based on predicted responses to specific therapies.
Smart cities: Optimizing urban infrastructure and services based on predicted usage patterns, from traffic flow to energy consumption.
Climate modeling: More accurate prediction of climate changes and extreme weather events to inform policy decisions and disaster preparedness.
Autonomous systems: Enabling self-driving vehicles and other autonomous systems to predict and respond to their environment in real-time.
Cybersecurity: Predicting and preventing security breaches before they occur by identifying patterns indicative of potential attacks.
Ethical Considerations
As predictive analytics becomes more powerful and pervasive, ethical considerations become increasingly important:
Bias and fairness: Ensuring models don't perpetuate or amplify existing biases in the data, which could lead to discriminatory outcomes.
Transparency: Making the basis for predictions clear and understandable to those affected by decisions based on predictive analytics.
Privacy: Balancing the benefits of prediction with individuals' right to privacy and control over their personal data.
Accountability: Establishing clear responsibility for the outcomes of predictive systems, especially when they inform critical decisions.
Human oversight: Maintaining appropriate human involvement in decision-making processes, particularly for high-stakes decisions.
Predictive analytics has fundamentally changed how decisions are made across industries. By applying predictive models, organizations can make smarter, more proactive decisions that reduce risks and optimize resource utilization. As data continues to grow in volume and importance, predictive analytics will become an increasingly essential tool for organizations looking to gain a competitive edge.
The ability to predict future events and trends is crucial in our complex, fast-paced world. From weekly weather forecasts to algorithm-enabled medical advancements, predictive analytics touches many aspects of modern life. By leveraging these powerful techniques, businesses and organizations can move beyond reactive decision-making to a more proactive approach that anticipates challenges and opportunities before they arise.
As we look to the future, the organizations that most effectively harness the power of predictive analytics will be best positioned to thrive in an increasingly data-driven world.
Predictive Analytics is the new buzz, nicely done.
Thank you